A new study from Vanderbilt University Medical Center finds that artificial intelligence can help improve and target the myriad of computerized alerts meant to aid doctors and other team members in their daily clinical decision-making. It proves the possibility.
These pop-up notifications advise users about everything from drug contraindications to gaps in patient care documentation. However, the exclusion criteria and targeting of these alerts are inadequate, with up to 90% being ignored, contributing to “alert fatigue.” From an information technology perspective, relying on human experts to solve targeting problems seems slow, expensive, and hit-or-miss.
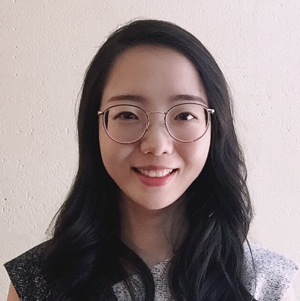
“Across healthcare, most of these well-intentioned automated alerts are overridden by busy users.Alerts serve an important purpose, but we all know they need to be improved. ” said lead author Dr. Siru Liu, assistant professor of biomedical informatics at VUMC.
Liu, lead author Adam Wright, Ph.D., professor of biomedical informatics and director of the Vanderbilt Center for Clinical Informatics, and the research team. Research February 22nd inside Journal of the American Medical Informatics Association.
Liu developed a machine learning approach to analyze two years of data on user and alert interactions at VUMC. Based on patient characteristics, the model accurately predicted when certain alerts would be ignored by users.
We then used a variety of processes and methods to look inside the predictive model, understand its reasoning, and generate suggestions for improving the alert logic. This step, called explainable artificial intelligence (AIX), involves converting the model's predictions into rules that explain when users are unlikely to accept an alert. for example, “if The patient is a hospice patient; after that Users are less likely to accept breast cancer screening warnings. ”
Of the 1,727 suggestions analyzed, 76 were found to be consistent with subsequent manual updates to VUMC alerts, and an additional 20 were found to be consistent with best practices determined through interviews with clinicians. did. The authors calculated that these 96 recommendations would eliminate 9.3% of the approximately 3 million alerts analyzed in the study, reducing disruptive pop-ups while maintaining patient safety.
“The alignment of the model's recommendations with the manual adjustments made by clinicians for alert logic highlights the strong potential of this technology to improve the quality and efficiency of healthcare,” Liu said. . “Our approach can identify areas missed by manual reviews and turn alert refinement into a continuous learning process.”
He added that the methodology not only improved alerts, but also uncovered situations that indicated workflow, education, and staffing issues. In this way, this approach has the potential to improve quality more broadly. “The transparency of our model reveals scenarios where alerts are ignored due to downstream issues beyond the alert itself.”
Liu and colleagues are considering several related projects, including a prospective multi-site study on the impact of machine learning for CDS improvement on patient care. Design an interface for CDS experts to visualize AIX processes and evaluate suggestions generated by the model. Based on user comments and current research literature he investigates the capabilities of large language models such as ChatGPT to optimize CDS alerts.
Other researchers working at VUMC include Allison McCoy, Ph.D., Josh Peterson, M.D., MPH, Thomas Lasko, M.D., Scott Nelson, M.S., Jennifer Andrews, M.D., and Lorraine Patterson, M.S.N. , Cheryl Cobb, MD, and David Mulherin. , PharmD, Colleen Morton, MD.
This research was supported by the National Institutes of Health (R00LM014097, R01AG062499, R01LM013995).